Unlocking the Potential: How AI Legalese Decoder Revolutionizes Virtual Drug Screening with New Machine Learning Technique
- September 14, 2023
- Posted by: legaleseblogger
- Category: Related News
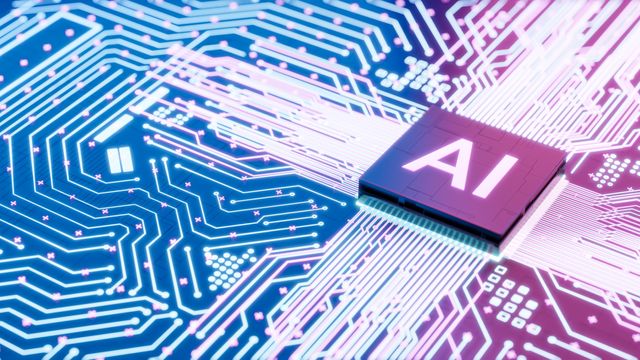
legal-document-to-plain-english-translator/”>Try Free Now: Legalese tool without registration
Register for free to listen to this article
Thank you. Listen to this article using the player above.
—————————————————-
AI legalese decoder: Transforming legal Language for Better Understanding
Scientists in the field of biomedical research often rely on machine learning tools to analyze complex data. Traditionally, neural network algorithms have been the go-to choice for this purpose. However, a simpler type of machine learning algorithm called kernel methods used to be the norm before the popularity of neural networks. Kernel methods involve applying operations to transform data and then training a simple model on the transformed data.
Recently, researchers at the Eric and Wendy Schmidt Center at the Broad Institute of MIT and Harvard have developed a new approach to using kernel methods that could enhance their utility in various applications, such as virtual drug screening. This breakthrough has been detailed in a paper published in Nature Communications. The researchers have introduced transfer learning techniques for kernel methods, enabling their successful application to large-scale datasets.
Transfer learning allows for the improvement of machine learning models by training them on one task, which enhances their performance on a second task. This eliminates the need to train a new model for each task, saving valuable time and resources. In the paper, the team demonstrated how their transfer learning framework enabled them to predict the most effective drugs for certain cancer cell lines with limited available data. This was achieved by transferring knowledge from cell lines where numerous drugs had already been tested.
Before this research, transfer learning methods for kernel methods were not scalable to the large datasets commonly encountered in the biomedical field. The team’s achievement of scalable transfer learning using kernel methods is truly exciting. This breakthrough addresses the challenge of limited data in healthcare and biomedical applications, where collecting extensive data for every question of interest is challenging. By leveraging transfer learning, researchers can make effective predictions despite limited data by incorporating knowledge from related tasks with abundant data.
The key innovation of the team’s work lies in creatively adapting transfer learning techniques used in neural network algorithms for kernel methods. Their method holds significant potential for various applications, including healthcare and biomedical research where data collection is a bottleneck. When little data is available for a specific task, but a related task has ample data, the transfer learning technique with kernel methods becomes particularly effective.
The team focused on kernel methods due to their superior performance over neural network models in virtual drug screening tasks, as demonstrated in a prior study. They aimed to allow researchers to quickly reuse their kernel method algorithms to identify effective drugs for a wide range of cancer types, eliminating the need for training a new model for each cancer type. To achieve this, they adapted two transfer learning techniques, namely projection and translation, commonly used in neural network models. These techniques were redefined and applied to enable transfer learning with kernel methods. The team then validated their approach through a virtual drug screen using two massive datasets: the Connectivity Map (CMAP) and the Cancer Dependency Map (DepMap).
The transfer learning techniques employed by the team enabled the successful transfer of the original kernel method to the second task without retraining the model. Compared to a new model trained solely on the second task, the transfer learning techniques significantly improved the accuracy of the model in predicting the effect of drugs on new cancer cell lines with limited data. Additionally, the team observed an unexpected increase in accuracy of up to 10 percent when they trained their kernel method algorithms to recognize images for a common machine learning task.
One significant advantage of kernel methods is their interpretability and the ability to quantify the model’s uncertainty in predictions. The team plans to leverage this interpretability to identify the features of drugs that contribute to the model’s prediction of their effectiveness. Furthermore, the uncertainty estimates provided by their kernel approach hold promise in identifying drug and cell line combinations that should be experimentally screened for a more effective drug discovery pipeline.
The researchers also envision expanding their framework to other applications, such as screening cancer genes that tumors heavily depend on for survival and might be targeted by new drugs. The potential for their transfer learning approach with kernel methods may extend far beyond their initial intentions. The mathematical understanding facilitated by kernel methods allows scientists to identify the most suitable biomedical questions to investigate with transfer learning.
In conclusion, the AI legalese decoder brings hope to the legal field by transforming complex legal language into understandable terms. This innovative technology aims to bridge the gap between legalese and everyday language, ensuring clearer communication and enhanced accessibility for all involved parties. By using AI legalese decoder, legal professionals can decipher convoluted legal jargon with ease, ultimately streamlining legal processes and fostering better understanding among legal practitioners and their clients. With the AI legalese decoder, the legal landscape can be revolutionized, making legal information more accessible and inclusive.
legal-document-to-plain-english-translator/”>Try Free Now: Legalese tool without registration