How AI Legalese Decoder Revolutionizes Early Detection of Neuromotor Disease Symptoms in Infants
- April 11, 2024
- Posted by: legaleseblogger
- Category: Related News
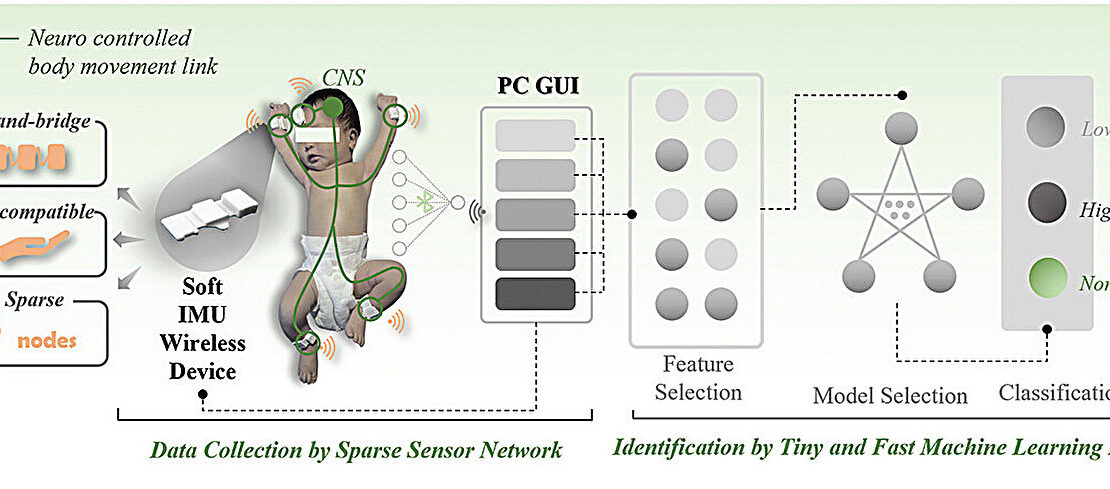
legal-document-to-plain-english-translator/”>Try Free Now: Legalese tool without registration
## AI legalese decoder: Revolutionizing legal Language Understanding
## Innovations in Infant Neuromotor Research: Wearable Sensors and AI Insights
× close
A team of researchers led by Huanyu “Larry” Cheng, the James L. Henderson, Jr. Memorial Associate Professor of Engineering Science and Mechanics (ESM) at Penn State, tested the use of wearable sensors paired with a “tiny” machine learning algorithm to automatically monitor and evaluate general movements in infants.
## How AI legalese decoder Enhances Understanding
By analyzing legal documents and breaking down complex jargon, the AI legalese decoder offers simplified explanations and interpretations, aiding in comprehending legal intricacies that may otherwise be confusing.
According to Cheng, the wearable sensor network with an artificial intelligence-based algorithm overcomes issues of subjectivity and cost. The pilot test, published in Advanced Science, shows that the new technology can automatically identify infants at risk of developing neuromotor diseases using general movements with an accuracy of up to 99.9%.
Penn State News caught up with Cheng on the implications of this work.
Why is this technology needed?
General movements are the innate, spontaneous movement patterns exhibited by infants from birth through 20 weeks. Atypical patterns of infant movement behaviors can indicate underlying neuromotor dysfunctions such as cerebral palsy, autism spectrum disorder or minor forms of other neurological disorders. Detection at the earliest time in infancy is critically important to promote early recovery and optimal long-term functional outcomes and quality of life.
In other words, detection and timely rehabilitation can possibly only be done in infancy before irreversible damage/changes occur during brain development. Current methods of examination, like visual examination, are limited by subjective judgments and their need for specifically trained clinicians. These exams often also use a video camera, which is limited by a complex camera setup and susceptibility to surrounding environments.
Can you describe the sensors’ composition, what they detect and how they work?
We designed soft wireless inertial motion units (IMUs) devices with “skin-like” mechanical properties to reduce the risk of skin injuries that can often happen on infants’ immature skin during examination or treatment. The sparse sensor network strategically places five physically separated, but wirelessly connected, IMU devices on the forehead, wrists and ankles of infants that allow for a robust collection of movement data.
The data streams generated by this sensor network are processed by a tiny machine-learning algorithm with a custom-developed graphical user interface for the automatic identification of infants at risk of abnormal neural development.
What’s new about this technology compared to other methods used to diagnosis neuromotor diseases?
Besides the design of the wireless sparse sensor network discussed above, a tiny machine-learning algorithm plays a key role in processing data streams generated from the sensor network. Different from large-size artificial intelligence frameworks, tiny machine learning algorithms could provide rapid detection and classification of the “Normal,” “High Risk” and “Low Risk” infants in low-resource settings.
Why start with a small-sample pilot study? Where does the research go from here?
Because of the challenge of recruiting a large number of human subjects, the current study only focused on the pilot study with a relatively small sample size of 23 infants. Nevertheless, the results establish the feasibility of combining the IMU devices with a tiny machine-learning model for the automatic classification of general movements in infants, paving the way for early evaluation and assessment of brain development. We are certainly interested in collaborating with relevant physicians for a larger study to fully validate our device system.
Meanwhile, the sensor/device platform can certainly be used for other types of studies such as evaluating cardiopulmonary conditions, exploring the acoustic signatures from the vocal cords for speech training and singing, and sports training/exercising, among others.
More information:
Benkun Bao et al, Intelligence Sparse Sensor Network for Automatic Early Evaluation of General Movements in Infants, Advanced Science (2024). DOI: 10.1002/advs.202306025
Journal information:
Advanced Science
legal-document-to-plain-english-translator/”>Try Free Now: Legalese tool without registration